AI for Sustainability: Why Intelligence Matters More Than Just Data
Jan 23, 2025



Table of Contents
Sustainability data is being generated across supply chains, facilities, and financial systems, but most enterprises struggle to convert it into meaningful action. Many AI solutions focus on collecting, structuring, and verifying sustainability metrics. While this is essential, static data alone does not drive operational improvements or emissions reductions at scale.
AI for Sustainability Must Go Beyond Data Collection
Traditional sustainability tools often provide historical analysis, but businesses need forward-looking intelligence to optimize operations, anticipate risks, and measure the impact of sustainability initiatives in real time. Net0 was designed with this in mind.
Our AI-first approach extends beyond data extraction and verification, providing deep analysis, forecasting, and decision-making capabilities that deliver measurable business outcomes.
This article explores how Net0’s AI differentiates itself by integrating both data infrastructure and intelligence to empower businesses with real-time, actionable sustainability insights. We will outline the key pillars of AI-driven sustainability, highlight the limitations of generic AI solutions, and showcase real-world applications where Net0 is delivering tangible results.
The Two Pillars of AI for Sustainability: Data Infrastructure & Data Intelligence
For AI to produce accurate, reliable sustainability insights, it must be built on a strong data foundation. Net0’s platform is structured around two key components:
A High-Integrity Data Infrastructure that ingests, standardizes, and secures sustainability data from multiple sources.
A Specialized AI Intelligence Layer that models emissions, forecasts trends, and optimizes operations in real time.
Building a Reliable Data Infrastructure
Sustainability data comes in many formats — structured (financial records, invoices, sensor logs) and unstructured (PDF reports, supplier disclosures). Traditional databases struggle to process such diverse datasets efficiently. Net0’s infrastructure is built for flexibility, incorporating:
Time-Series Databases for tracking emissions fluctuations over time, enabling anomaly detection and trend forecasting.
Graph Databases to map relationships across supply chains, linking emissions data with procurement decisions.
Data Lakes for ingesting vast amounts of structured and unstructured sustainability data, supporting machine learning model training.
Document Stores that automatically extract key ESG data from invoices, regulatory reports, and supplier disclosures.
Message Queues & Streaming Pipelines that facilitate real-time data ingestion from IoT sensors, external databases, and enterprise systems.
By combining these architectures, Net0 ensures scalability, accuracy, and adaptability—allowing businesses to track sustainability data without performance bottlenecks.
From Data to Intelligence: Enabling Proactive Decision-Making
Now that we know - raw data alone does not enable action. Net0’s AI models process sustainability data dynamically, turning raw inputs into real-time insights that drive measurable sustainability outcomes. Whether it’s forecasting emissions, optimizing resource use, or ensuring compliance, AI-powered intelligence is what transforms sustainability from a reporting function into a business driver.
AI for Data Analysis
Collecting and structuring data is important, but if the data is incomplete, inconsistent, or misleading, businesses risk making the wrong choices. Net0’s AI continuously analyzes and refines data quality to ensure accuracy, detect anomalies, and fill in missing gaps with machine learning predictions.
To achieve this, our AI is structured into specialized categories, each designed for a specific function:
Predictive modeling systems analyze sustainability trends and emissions patterns. For example, a retailer tracking its carbon footprint across thousands of stores can use AI to forecast future emissions hotspots and optimize energy consumption before regulatory deadlines.
Scenario simulation models anticipate risks from regulatory shifts, supply chain disruptions, and operational constraints. A global manufacturer, for instance, can model the impact of stricter EU emissions policies on its production sites and adjust strategies accordingly.
Optimization algorithms refine sustainability strategies by continuously learning from new data. An energy-intensive industrial facility can use AI-driven real-time adjustments to reduce peak-time electricity demand, lowering both costs and emissions.
Document processing and NLP models extract insights from sustainability reports, ESG disclosures, and regulatory filings, automatically categorizing and summarizing key compliance data for reporting teams.
Real-time sensor data analysis processes IoT inputs from factories, offices, and logistics networks, enabling businesses to identify inefficiencies in energy use, waste management, and air quality before they become major issues.
Decarbonization AI
Decarbonization it’s about making smart decisions to reduce emissions efficiently. Net0’s AI enables businesses to implement the right carbon reduction strategies at the right time.
Emissions modeling frameworks provide highly accurate Scope 1, 2, and 3 calculations, helping enterprises pinpoint their largest carbon sources. A multinational retailer, for instance, can use AI to compare emissions from different suppliers, selecting the most sustainable sourcing options without affecting supply chain efficiency.
Optimization engines dynamically adjust decarbonization strategies based on real-time data. A logistics company can use AI to reroute delivery fleets based on emissions impact rather than just distance, lowering fuel consumption and carbon output.
Impact assessment models quantify the financial and operational effects of sustainability initiatives, ensuring that investments in green technologies, renewables, or energy efficiency upgrades are measured against cost savings and business goals. A manufacturing company, for example, can use AI to assess whether switching to alternative materials will reduce emissions while maintaining product quality and profitability.
ESG prioritization tools align sustainability actions with business objectives, helping enterprises decide where to allocate resources for maximum emissions reduction with minimal financial disruption.
Reporting AI
Sustainability reporting requires transparency and trust. With Net0’s AI, enterprises can automate ESG and sustainability disclosures and gain insights beyond what traditional reporting tools offer.
Automated report generation transforms unstructured data into structured ESG disclosures, reducing reporting workloads by up to 90%. For example, a global fashion brand struggling to consolidate supplier emissions data across multiple regions can use Net0’s AI to automatically extract emissions metrics from supplier invoices and generate compliant CSRD and SEC reports in minutes.
Regulatory trend analysis continuously scans for changes in frameworks such as CSRD, SEC, and IFRS, alerting companies to new compliance requirements before they become an issue. A financial institution, for instance, can rely on AI to track evolving sustainable finance regulations and adjust ESG investment disclosures accordingly.
Related content
Explore how AI can drive sustainability in your business with these insights:• Article: Why Artificial Intelligence Is Essential When Tackling Climate Change
• Article: 5 Reasons Net0 Builds Its Own AI Models
• Article: Redefining the Landscape: How AI Transforms Carbon Data Collection for Effective Emissions Management
Why Generic AI Fails to Deliver Sustainability Intelligence
Most AI solutions in the market were not built with sustainability in mind. They rely on generalized machine learning models designed for broad applications, making them ill-equipped to handle the complexity of sustainability data. From emissions calculations to ESG reporting and supply chain optimization, sustainability demands specialized AI models that can interpret, adapt, and act in real-time. However, generic AI solutions often fall short in three key areas.
1. Lack of Context-Aware Models
A major flaw of generic AI is its inability to understand the nuances of sustainability data. Standard models trained on financial or operational datasets lack sustainability-specific logic, leading to inaccuracies when analyzing emissions, resource efficiency, or regulatory frameworks.
For example, Scope 3 emissions tracking requires AI to process data from suppliers, logistics providers, and operational sites, each with different reporting formats and varying levels of data availability. A generalized AI model might misclassify emissions data, failing to recognize the difference between direct and indirect emissions or inaccurately linking a company’s carbon footprint to supplier activities.
Similarly, sustainability regulations differ across regions, but most AI platforms do not account for jurisdiction-specific requirements. A global company may need to comply with the SEC’s climate disclosure rules in the U.S., CSRD in Europe, and UAE’s ESG reporting mandates—all of which have different thresholds, categories, and reporting standards. Without context-aware AI, businesses risk misreporting their sustainability data and facing compliance failures.
2. Static Insights Instead of Real-Time Decision-Making
Sustainability is not static—regulations evolve, supply chains shift, and operational efficiencies fluctuate. Yet, many AI solutions operate on pre-trained models that do not update dynamically. These models fail to adapt to new emissions factors, evolving reporting requirements, or sudden supply chain disruptions, leaving businesses reactive rather than proactive.
For instance, a manufacturer might introduce a new energy-efficient production line to cut emissions. A static AI model would still rely on historical emissions data, delaying insights that could inform immediate adjustments to carbon reduction targets. Similarly, if an energy provider suddenly increases the share of renewables in the grid mix, a company using a static AI model would continue calculating emissions based on outdated energy assumptions, leading to inaccurate carbon reporting.
In contrast, Net0’s AI continuously retrains itself using live operational data. By incorporating real-time sensor readings, updated emissions factors, and the latest regulatory changes, it enables enterprises to adjust sustainability strategies dynamically rather than waiting for outdated reports.
3. Data Security & Compliance Risks
Sustainability data is deeply interconnected with financial disclosures, supplier contracts, and operational records, making it highly sensitive. Companies handling ESG and emissions data must ensure that it is securely stored, processed, and shared in compliance with regional data protection laws. However, many AI solutions lack enterprise-grade security frameworks, leaving businesses vulnerable to data breaches, compliance violations, and regulatory penalties.
A company operating across multiple regions may be required to store its sustainability data locally due to data sovereignty laws — but most AI platforms do not offer flexible deployment options. This leads to compliance risks, as businesses must choose between using non-compliant cloud-based AI models or manually segregating data across different systems.
Net0 addresses these risks by providing:
Full data sovereignty with cloud, on-premise, and hybrid deployment options, allowing enterprises to meet jurisdictional compliance requirements.
Enterprise-grade encryption and role-based access controls, ensuring sustainability data is protected from unauthorized access.
Automated compliance tracking, alerting businesses to changes in data protection laws that impact their ESG and emissions reporting processes.
The Future of AI-Driven Sustainability: Adaptive, Predictive, Actionable
The future of sustainability AI lies in its ability to evolve alongside the businesses it serves. AI must be dynamic, continuously learning, and directly influencing decision-making.
Net0’s AI is built to be adaptive, processing live sustainability data across various domains - emissions, energy, waste, water, and beyond - to adjust strategies in real time.
It is predictive, using advanced modeling to anticipate risks, forecast trends, and suggest the most efficient sustainability pathways before issues arise.
And most importantly, it is actionable, providing enterprises with clear, data-backed recommendations that directly influence operational efficiency and strategic planning.
By embedding AI-driven intelligence into sustainability management, businesses can transition from compliance-focused efforts to long-term value creation. Enterprises that leverage AI for sustainability will not only reduce risks but also uncover opportunities for cost savings, innovation, and competitive differentiation.
Final Thoughts
Net0’s AI transforms sustainability data into action. By integrating real-time insights across emissions, energy, waste, supply chains, and operational efficiency, it enables businesses to make sustainability a core driver of profitability and growth.
Net0’s platform combines high-integrity data infrastructure with real-time decision-making models, ensuring that enterprises can optimize sustainability efforts at scale.
Let’s discuss how Net0’s AI can enhance your sustainability strategy.
Sustainability data is being generated across supply chains, facilities, and financial systems, but most enterprises struggle to convert it into meaningful action. Many AI solutions focus on collecting, structuring, and verifying sustainability metrics. While this is essential, static data alone does not drive operational improvements or emissions reductions at scale.
AI for Sustainability Must Go Beyond Data Collection
Traditional sustainability tools often provide historical analysis, but businesses need forward-looking intelligence to optimize operations, anticipate risks, and measure the impact of sustainability initiatives in real time. Net0 was designed with this in mind.
Our AI-first approach extends beyond data extraction and verification, providing deep analysis, forecasting, and decision-making capabilities that deliver measurable business outcomes.
This article explores how Net0’s AI differentiates itself by integrating both data infrastructure and intelligence to empower businesses with real-time, actionable sustainability insights. We will outline the key pillars of AI-driven sustainability, highlight the limitations of generic AI solutions, and showcase real-world applications where Net0 is delivering tangible results.
The Two Pillars of AI for Sustainability: Data Infrastructure & Data Intelligence
For AI to produce accurate, reliable sustainability insights, it must be built on a strong data foundation. Net0’s platform is structured around two key components:
A High-Integrity Data Infrastructure that ingests, standardizes, and secures sustainability data from multiple sources.
A Specialized AI Intelligence Layer that models emissions, forecasts trends, and optimizes operations in real time.
Building a Reliable Data Infrastructure
Sustainability data comes in many formats — structured (financial records, invoices, sensor logs) and unstructured (PDF reports, supplier disclosures). Traditional databases struggle to process such diverse datasets efficiently. Net0’s infrastructure is built for flexibility, incorporating:
Time-Series Databases for tracking emissions fluctuations over time, enabling anomaly detection and trend forecasting.
Graph Databases to map relationships across supply chains, linking emissions data with procurement decisions.
Data Lakes for ingesting vast amounts of structured and unstructured sustainability data, supporting machine learning model training.
Document Stores that automatically extract key ESG data from invoices, regulatory reports, and supplier disclosures.
Message Queues & Streaming Pipelines that facilitate real-time data ingestion from IoT sensors, external databases, and enterprise systems.
By combining these architectures, Net0 ensures scalability, accuracy, and adaptability—allowing businesses to track sustainability data without performance bottlenecks.
From Data to Intelligence: Enabling Proactive Decision-Making
Now that we know - raw data alone does not enable action. Net0’s AI models process sustainability data dynamically, turning raw inputs into real-time insights that drive measurable sustainability outcomes. Whether it’s forecasting emissions, optimizing resource use, or ensuring compliance, AI-powered intelligence is what transforms sustainability from a reporting function into a business driver.
AI for Data Analysis
Collecting and structuring data is important, but if the data is incomplete, inconsistent, or misleading, businesses risk making the wrong choices. Net0’s AI continuously analyzes and refines data quality to ensure accuracy, detect anomalies, and fill in missing gaps with machine learning predictions.
To achieve this, our AI is structured into specialized categories, each designed for a specific function:
Predictive modeling systems analyze sustainability trends and emissions patterns. For example, a retailer tracking its carbon footprint across thousands of stores can use AI to forecast future emissions hotspots and optimize energy consumption before regulatory deadlines.
Scenario simulation models anticipate risks from regulatory shifts, supply chain disruptions, and operational constraints. A global manufacturer, for instance, can model the impact of stricter EU emissions policies on its production sites and adjust strategies accordingly.
Optimization algorithms refine sustainability strategies by continuously learning from new data. An energy-intensive industrial facility can use AI-driven real-time adjustments to reduce peak-time electricity demand, lowering both costs and emissions.
Document processing and NLP models extract insights from sustainability reports, ESG disclosures, and regulatory filings, automatically categorizing and summarizing key compliance data for reporting teams.
Real-time sensor data analysis processes IoT inputs from factories, offices, and logistics networks, enabling businesses to identify inefficiencies in energy use, waste management, and air quality before they become major issues.
Decarbonization AI
Decarbonization it’s about making smart decisions to reduce emissions efficiently. Net0’s AI enables businesses to implement the right carbon reduction strategies at the right time.
Emissions modeling frameworks provide highly accurate Scope 1, 2, and 3 calculations, helping enterprises pinpoint their largest carbon sources. A multinational retailer, for instance, can use AI to compare emissions from different suppliers, selecting the most sustainable sourcing options without affecting supply chain efficiency.
Optimization engines dynamically adjust decarbonization strategies based on real-time data. A logistics company can use AI to reroute delivery fleets based on emissions impact rather than just distance, lowering fuel consumption and carbon output.
Impact assessment models quantify the financial and operational effects of sustainability initiatives, ensuring that investments in green technologies, renewables, or energy efficiency upgrades are measured against cost savings and business goals. A manufacturing company, for example, can use AI to assess whether switching to alternative materials will reduce emissions while maintaining product quality and profitability.
ESG prioritization tools align sustainability actions with business objectives, helping enterprises decide where to allocate resources for maximum emissions reduction with minimal financial disruption.
Reporting AI
Sustainability reporting requires transparency and trust. With Net0’s AI, enterprises can automate ESG and sustainability disclosures and gain insights beyond what traditional reporting tools offer.
Automated report generation transforms unstructured data into structured ESG disclosures, reducing reporting workloads by up to 90%. For example, a global fashion brand struggling to consolidate supplier emissions data across multiple regions can use Net0’s AI to automatically extract emissions metrics from supplier invoices and generate compliant CSRD and SEC reports in minutes.
Regulatory trend analysis continuously scans for changes in frameworks such as CSRD, SEC, and IFRS, alerting companies to new compliance requirements before they become an issue. A financial institution, for instance, can rely on AI to track evolving sustainable finance regulations and adjust ESG investment disclosures accordingly.
Related content
Explore how AI can drive sustainability in your business with these insights:• Article: Why Artificial Intelligence Is Essential When Tackling Climate Change
• Article: 5 Reasons Net0 Builds Its Own AI Models
• Article: Redefining the Landscape: How AI Transforms Carbon Data Collection for Effective Emissions Management
Why Generic AI Fails to Deliver Sustainability Intelligence
Most AI solutions in the market were not built with sustainability in mind. They rely on generalized machine learning models designed for broad applications, making them ill-equipped to handle the complexity of sustainability data. From emissions calculations to ESG reporting and supply chain optimization, sustainability demands specialized AI models that can interpret, adapt, and act in real-time. However, generic AI solutions often fall short in three key areas.
1. Lack of Context-Aware Models
A major flaw of generic AI is its inability to understand the nuances of sustainability data. Standard models trained on financial or operational datasets lack sustainability-specific logic, leading to inaccuracies when analyzing emissions, resource efficiency, or regulatory frameworks.
For example, Scope 3 emissions tracking requires AI to process data from suppliers, logistics providers, and operational sites, each with different reporting formats and varying levels of data availability. A generalized AI model might misclassify emissions data, failing to recognize the difference between direct and indirect emissions or inaccurately linking a company’s carbon footprint to supplier activities.
Similarly, sustainability regulations differ across regions, but most AI platforms do not account for jurisdiction-specific requirements. A global company may need to comply with the SEC’s climate disclosure rules in the U.S., CSRD in Europe, and UAE’s ESG reporting mandates—all of which have different thresholds, categories, and reporting standards. Without context-aware AI, businesses risk misreporting their sustainability data and facing compliance failures.
2. Static Insights Instead of Real-Time Decision-Making
Sustainability is not static—regulations evolve, supply chains shift, and operational efficiencies fluctuate. Yet, many AI solutions operate on pre-trained models that do not update dynamically. These models fail to adapt to new emissions factors, evolving reporting requirements, or sudden supply chain disruptions, leaving businesses reactive rather than proactive.
For instance, a manufacturer might introduce a new energy-efficient production line to cut emissions. A static AI model would still rely on historical emissions data, delaying insights that could inform immediate adjustments to carbon reduction targets. Similarly, if an energy provider suddenly increases the share of renewables in the grid mix, a company using a static AI model would continue calculating emissions based on outdated energy assumptions, leading to inaccurate carbon reporting.
In contrast, Net0’s AI continuously retrains itself using live operational data. By incorporating real-time sensor readings, updated emissions factors, and the latest regulatory changes, it enables enterprises to adjust sustainability strategies dynamically rather than waiting for outdated reports.
3. Data Security & Compliance Risks
Sustainability data is deeply interconnected with financial disclosures, supplier contracts, and operational records, making it highly sensitive. Companies handling ESG and emissions data must ensure that it is securely stored, processed, and shared in compliance with regional data protection laws. However, many AI solutions lack enterprise-grade security frameworks, leaving businesses vulnerable to data breaches, compliance violations, and regulatory penalties.
A company operating across multiple regions may be required to store its sustainability data locally due to data sovereignty laws — but most AI platforms do not offer flexible deployment options. This leads to compliance risks, as businesses must choose between using non-compliant cloud-based AI models or manually segregating data across different systems.
Net0 addresses these risks by providing:
Full data sovereignty with cloud, on-premise, and hybrid deployment options, allowing enterprises to meet jurisdictional compliance requirements.
Enterprise-grade encryption and role-based access controls, ensuring sustainability data is protected from unauthorized access.
Automated compliance tracking, alerting businesses to changes in data protection laws that impact their ESG and emissions reporting processes.
The Future of AI-Driven Sustainability: Adaptive, Predictive, Actionable
The future of sustainability AI lies in its ability to evolve alongside the businesses it serves. AI must be dynamic, continuously learning, and directly influencing decision-making.
Net0’s AI is built to be adaptive, processing live sustainability data across various domains - emissions, energy, waste, water, and beyond - to adjust strategies in real time.
It is predictive, using advanced modeling to anticipate risks, forecast trends, and suggest the most efficient sustainability pathways before issues arise.
And most importantly, it is actionable, providing enterprises with clear, data-backed recommendations that directly influence operational efficiency and strategic planning.
By embedding AI-driven intelligence into sustainability management, businesses can transition from compliance-focused efforts to long-term value creation. Enterprises that leverage AI for sustainability will not only reduce risks but also uncover opportunities for cost savings, innovation, and competitive differentiation.
Final Thoughts
Net0’s AI transforms sustainability data into action. By integrating real-time insights across emissions, energy, waste, supply chains, and operational efficiency, it enables businesses to make sustainability a core driver of profitability and growth.
Net0’s platform combines high-integrity data infrastructure with real-time decision-making models, ensuring that enterprises can optimize sustainability efforts at scale.
Let’s discuss how Net0’s AI can enhance your sustainability strategy.
Sustainability data is being generated across supply chains, facilities, and financial systems, but most enterprises struggle to convert it into meaningful action. Many AI solutions focus on collecting, structuring, and verifying sustainability metrics. While this is essential, static data alone does not drive operational improvements or emissions reductions at scale.
AI for Sustainability Must Go Beyond Data Collection
Traditional sustainability tools often provide historical analysis, but businesses need forward-looking intelligence to optimize operations, anticipate risks, and measure the impact of sustainability initiatives in real time. Net0 was designed with this in mind.
Our AI-first approach extends beyond data extraction and verification, providing deep analysis, forecasting, and decision-making capabilities that deliver measurable business outcomes.
This article explores how Net0’s AI differentiates itself by integrating both data infrastructure and intelligence to empower businesses with real-time, actionable sustainability insights. We will outline the key pillars of AI-driven sustainability, highlight the limitations of generic AI solutions, and showcase real-world applications where Net0 is delivering tangible results.
The Two Pillars of AI for Sustainability: Data Infrastructure & Data Intelligence
For AI to produce accurate, reliable sustainability insights, it must be built on a strong data foundation. Net0’s platform is structured around two key components:
A High-Integrity Data Infrastructure that ingests, standardizes, and secures sustainability data from multiple sources.
A Specialized AI Intelligence Layer that models emissions, forecasts trends, and optimizes operations in real time.
Building a Reliable Data Infrastructure
Sustainability data comes in many formats — structured (financial records, invoices, sensor logs) and unstructured (PDF reports, supplier disclosures). Traditional databases struggle to process such diverse datasets efficiently. Net0’s infrastructure is built for flexibility, incorporating:
Time-Series Databases for tracking emissions fluctuations over time, enabling anomaly detection and trend forecasting.
Graph Databases to map relationships across supply chains, linking emissions data with procurement decisions.
Data Lakes for ingesting vast amounts of structured and unstructured sustainability data, supporting machine learning model training.
Document Stores that automatically extract key ESG data from invoices, regulatory reports, and supplier disclosures.
Message Queues & Streaming Pipelines that facilitate real-time data ingestion from IoT sensors, external databases, and enterprise systems.
By combining these architectures, Net0 ensures scalability, accuracy, and adaptability—allowing businesses to track sustainability data without performance bottlenecks.
From Data to Intelligence: Enabling Proactive Decision-Making
Now that we know - raw data alone does not enable action. Net0’s AI models process sustainability data dynamically, turning raw inputs into real-time insights that drive measurable sustainability outcomes. Whether it’s forecasting emissions, optimizing resource use, or ensuring compliance, AI-powered intelligence is what transforms sustainability from a reporting function into a business driver.
AI for Data Analysis
Collecting and structuring data is important, but if the data is incomplete, inconsistent, or misleading, businesses risk making the wrong choices. Net0’s AI continuously analyzes and refines data quality to ensure accuracy, detect anomalies, and fill in missing gaps with machine learning predictions.
To achieve this, our AI is structured into specialized categories, each designed for a specific function:
Predictive modeling systems analyze sustainability trends and emissions patterns. For example, a retailer tracking its carbon footprint across thousands of stores can use AI to forecast future emissions hotspots and optimize energy consumption before regulatory deadlines.
Scenario simulation models anticipate risks from regulatory shifts, supply chain disruptions, and operational constraints. A global manufacturer, for instance, can model the impact of stricter EU emissions policies on its production sites and adjust strategies accordingly.
Optimization algorithms refine sustainability strategies by continuously learning from new data. An energy-intensive industrial facility can use AI-driven real-time adjustments to reduce peak-time electricity demand, lowering both costs and emissions.
Document processing and NLP models extract insights from sustainability reports, ESG disclosures, and regulatory filings, automatically categorizing and summarizing key compliance data for reporting teams.
Real-time sensor data analysis processes IoT inputs from factories, offices, and logistics networks, enabling businesses to identify inefficiencies in energy use, waste management, and air quality before they become major issues.
Decarbonization AI
Decarbonization it’s about making smart decisions to reduce emissions efficiently. Net0’s AI enables businesses to implement the right carbon reduction strategies at the right time.
Emissions modeling frameworks provide highly accurate Scope 1, 2, and 3 calculations, helping enterprises pinpoint their largest carbon sources. A multinational retailer, for instance, can use AI to compare emissions from different suppliers, selecting the most sustainable sourcing options without affecting supply chain efficiency.
Optimization engines dynamically adjust decarbonization strategies based on real-time data. A logistics company can use AI to reroute delivery fleets based on emissions impact rather than just distance, lowering fuel consumption and carbon output.
Impact assessment models quantify the financial and operational effects of sustainability initiatives, ensuring that investments in green technologies, renewables, or energy efficiency upgrades are measured against cost savings and business goals. A manufacturing company, for example, can use AI to assess whether switching to alternative materials will reduce emissions while maintaining product quality and profitability.
ESG prioritization tools align sustainability actions with business objectives, helping enterprises decide where to allocate resources for maximum emissions reduction with minimal financial disruption.
Reporting AI
Sustainability reporting requires transparency and trust. With Net0’s AI, enterprises can automate ESG and sustainability disclosures and gain insights beyond what traditional reporting tools offer.
Automated report generation transforms unstructured data into structured ESG disclosures, reducing reporting workloads by up to 90%. For example, a global fashion brand struggling to consolidate supplier emissions data across multiple regions can use Net0’s AI to automatically extract emissions metrics from supplier invoices and generate compliant CSRD and SEC reports in minutes.
Regulatory trend analysis continuously scans for changes in frameworks such as CSRD, SEC, and IFRS, alerting companies to new compliance requirements before they become an issue. A financial institution, for instance, can rely on AI to track evolving sustainable finance regulations and adjust ESG investment disclosures accordingly.
Related content
Explore how AI can drive sustainability in your business with these insights:• Article: Why Artificial Intelligence Is Essential When Tackling Climate Change
• Article: 5 Reasons Net0 Builds Its Own AI Models
• Article: Redefining the Landscape: How AI Transforms Carbon Data Collection for Effective Emissions Management
Why Generic AI Fails to Deliver Sustainability Intelligence
Most AI solutions in the market were not built with sustainability in mind. They rely on generalized machine learning models designed for broad applications, making them ill-equipped to handle the complexity of sustainability data. From emissions calculations to ESG reporting and supply chain optimization, sustainability demands specialized AI models that can interpret, adapt, and act in real-time. However, generic AI solutions often fall short in three key areas.
1. Lack of Context-Aware Models
A major flaw of generic AI is its inability to understand the nuances of sustainability data. Standard models trained on financial or operational datasets lack sustainability-specific logic, leading to inaccuracies when analyzing emissions, resource efficiency, or regulatory frameworks.
For example, Scope 3 emissions tracking requires AI to process data from suppliers, logistics providers, and operational sites, each with different reporting formats and varying levels of data availability. A generalized AI model might misclassify emissions data, failing to recognize the difference between direct and indirect emissions or inaccurately linking a company’s carbon footprint to supplier activities.
Similarly, sustainability regulations differ across regions, but most AI platforms do not account for jurisdiction-specific requirements. A global company may need to comply with the SEC’s climate disclosure rules in the U.S., CSRD in Europe, and UAE’s ESG reporting mandates—all of which have different thresholds, categories, and reporting standards. Without context-aware AI, businesses risk misreporting their sustainability data and facing compliance failures.
2. Static Insights Instead of Real-Time Decision-Making
Sustainability is not static—regulations evolve, supply chains shift, and operational efficiencies fluctuate. Yet, many AI solutions operate on pre-trained models that do not update dynamically. These models fail to adapt to new emissions factors, evolving reporting requirements, or sudden supply chain disruptions, leaving businesses reactive rather than proactive.
For instance, a manufacturer might introduce a new energy-efficient production line to cut emissions. A static AI model would still rely on historical emissions data, delaying insights that could inform immediate adjustments to carbon reduction targets. Similarly, if an energy provider suddenly increases the share of renewables in the grid mix, a company using a static AI model would continue calculating emissions based on outdated energy assumptions, leading to inaccurate carbon reporting.
In contrast, Net0’s AI continuously retrains itself using live operational data. By incorporating real-time sensor readings, updated emissions factors, and the latest regulatory changes, it enables enterprises to adjust sustainability strategies dynamically rather than waiting for outdated reports.
3. Data Security & Compliance Risks
Sustainability data is deeply interconnected with financial disclosures, supplier contracts, and operational records, making it highly sensitive. Companies handling ESG and emissions data must ensure that it is securely stored, processed, and shared in compliance with regional data protection laws. However, many AI solutions lack enterprise-grade security frameworks, leaving businesses vulnerable to data breaches, compliance violations, and regulatory penalties.
A company operating across multiple regions may be required to store its sustainability data locally due to data sovereignty laws — but most AI platforms do not offer flexible deployment options. This leads to compliance risks, as businesses must choose between using non-compliant cloud-based AI models or manually segregating data across different systems.
Net0 addresses these risks by providing:
Full data sovereignty with cloud, on-premise, and hybrid deployment options, allowing enterprises to meet jurisdictional compliance requirements.
Enterprise-grade encryption and role-based access controls, ensuring sustainability data is protected from unauthorized access.
Automated compliance tracking, alerting businesses to changes in data protection laws that impact their ESG and emissions reporting processes.
The Future of AI-Driven Sustainability: Adaptive, Predictive, Actionable
The future of sustainability AI lies in its ability to evolve alongside the businesses it serves. AI must be dynamic, continuously learning, and directly influencing decision-making.
Net0’s AI is built to be adaptive, processing live sustainability data across various domains - emissions, energy, waste, water, and beyond - to adjust strategies in real time.
It is predictive, using advanced modeling to anticipate risks, forecast trends, and suggest the most efficient sustainability pathways before issues arise.
And most importantly, it is actionable, providing enterprises with clear, data-backed recommendations that directly influence operational efficiency and strategic planning.
By embedding AI-driven intelligence into sustainability management, businesses can transition from compliance-focused efforts to long-term value creation. Enterprises that leverage AI for sustainability will not only reduce risks but also uncover opportunities for cost savings, innovation, and competitive differentiation.
Final Thoughts
Net0’s AI transforms sustainability data into action. By integrating real-time insights across emissions, energy, waste, supply chains, and operational efficiency, it enables businesses to make sustainability a core driver of profitability and growth.
Net0’s platform combines high-integrity data infrastructure with real-time decision-making models, ensuring that enterprises can optimize sustainability efforts at scale.
Let’s discuss how Net0’s AI can enhance your sustainability strategy.
Written by
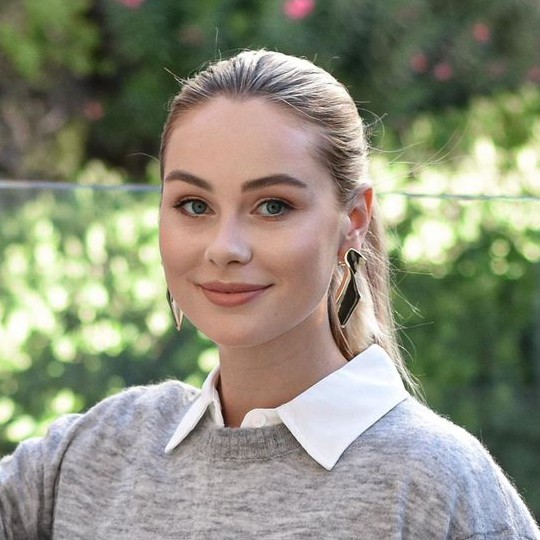
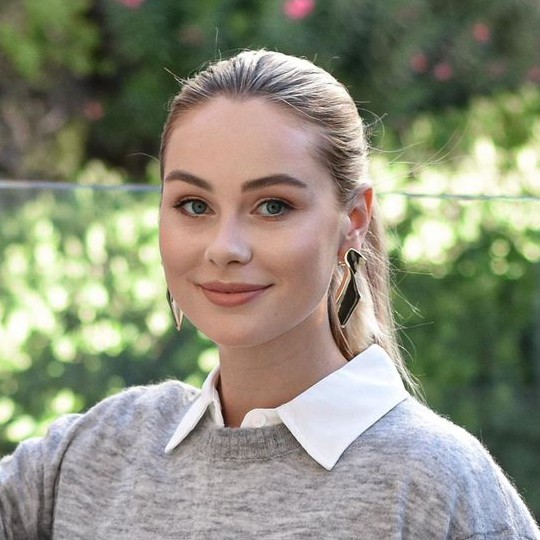
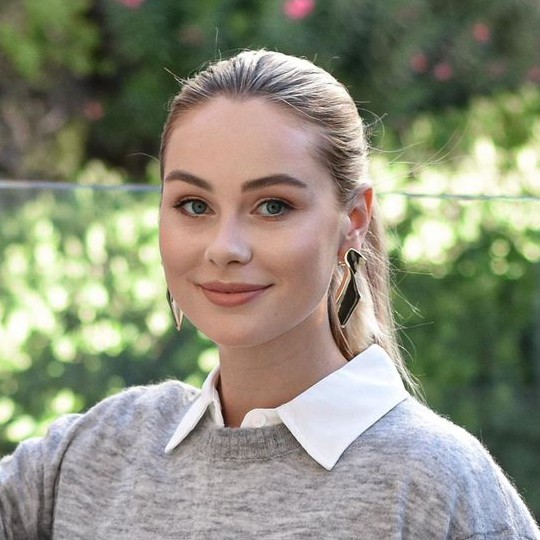
Sofia Fominova
As Co-Founder of Net0, Sofia Fominova helps enterprises and governments leverage AI and data to achieve their sustainability goals. With over 60 AI-powered solutions, Net0 provides end-to-end sustainability tools—covering everything from automated data collection and full carbon management to industry-specific solutions for waste, water, and beyond. By addressing sustainability at every level, Net0 enables organizations to drive efficiency, reduce environmental impact, and create lasting change.
Reimagine Sustainability
with AI
Capitalize on the economic opportunities of sustainability with Net0's emissions management software.

Reimagine Sustainability
with AI
Capitalize on the economic opportunities of sustainability with Net0's emissions management software.

Reimagine Sustainability
with AI
Capitalize on the economic opportunities of sustainability with Net0's emissions management software.

Reimagine Sustainability
with AI
Capitalize on the economic opportunities of sustainability with Net0's emissions management software.
